YOUR CONTACT
This Page Could Not Be Found
The reason for this could be that you have called up an outdated URL or we have archived or renamed the page in question.
But have a look at our homepage. Perhaps you will find the content you are looking for there.
We are also happy to help you with direct enquiries. Please use the contact data provided below.
Your Contact for more information
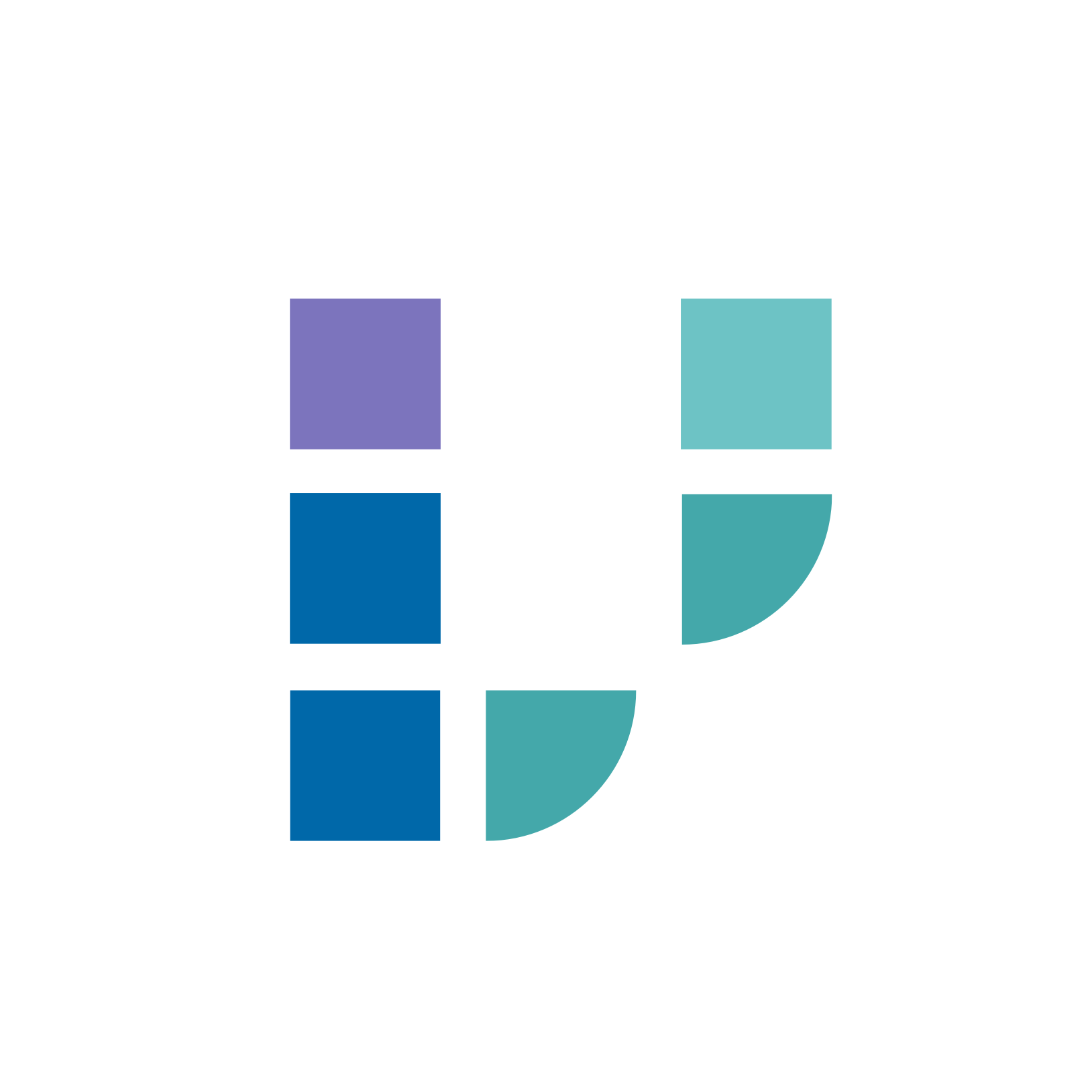
Vidispine - An Arvato Systems Brand